
Curiosity Lab
Acerca de
Curious Data Scientist
In the process of building machine learning models data sometimes must be sampled before the learning process can be applied. This step, known as instance selection, is mostly done to reduce the amount of data in a volume that will allow the computing resources required for the learning phase to be reduced. In addition, it also removes noisy data that can affect the learning quality. While the two objectives are often in conflict, in most current approaches, it is impossible to control the balance between them. We propose a reinforcement learning-based approach for instance selection, called curious instance selection (CIS), which evaluates clusters of instances using the curiosity loop architecture. The output of the algorithm is a matrix that represents the value of adding a cluster of instances to existing instances. This matrix enables the computation of the Pareto front and demonstrates the ability to balance the noise and volume reduction objectives. CIS was evaluated on five datasets, and its performance was compared with the performance of three state-of-the-art algorithms. Our results show that CIS not only provides enhanced flexibility but also achieves higher effectiveness (reduction times accuracy). This approach strengthens the appeal of using curiosity-based algorithms in data science. to the full article

In state-of-the-art big-data applications, the process of building machine learning models can be very challenging due to continuous changes in data structures and the need for human interaction to tune the variables and models over time. Hence, expedited learning in rapidly changing environments is required. In this work, we address this challenge by implementing concepts from the field of intrinsically motivated computational learning, also known as artificial curiosity (AC). In AC, an autonomous agent acts to optimize its learning about itself and its environment by receiving internal rewards based on prediction errors. We present a novel method of intrinsically motivated learning, based on the curiosity loop, to learn the data structures in large and varied datasets. An autonomous agent learns to select a subset of relevant features in the data, i.e., feature selection, to be used later for model construction. The agent optimizes its learning about the data structure over time without requiring external supervision. We show that our method, called the Curious Feature Selection (CFS) algorithm, positively impacts the accuracy of learning models on three public datasets. to the full article
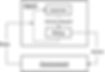